How To Ensure Robust Data Collection for Accurate Insights

In today’s age of data, robust data collection is key for gaining insights that support decision making. Organizations that focus on effective gathering improve their analytical capabilities and stand out from competitors. This post will show best practices and techniques for robust data collection, using modern methods and tools.
By knowing the important parts of a good governance framework for data and the need for monitoring, you will see how to keep your data's quality. Whether you are an expert analyst or just beginning, this overview will give you tools and strategies to change raw data into useful insights. Let’s explore and discover the potential of your data with robust collection methods!
Best Practices for Robust Data Collection
Robust data collection helps create base for accurate insights and solid analytical strategies. A strong data collection process cut errors down and improves data quality. To trust insights, data should reflect reality. Case studies show inconsistency in data collection can lead to a loss in actionable insights, as much as 30%.
A key practice for robust data collection is use of standard data entry processes. With a uniform method for data capture, organizations reduce errors from different input variations. In data analytics, standard practices can cut data entry errors, by 25%. This can mean clear guidelines on acceptable formats and training team members on these standards.
Utilizing automated tools for data collection is another effective method. Automation not only boosts efficiency but it also keeps data consistent. Tools that link with other systems help cut human-errors risks and keep data integrity. Studies say that organizations using automated data collection see improvement by 20% in accuracy compared to manual methods.
A vital part of robust data collection is regular data audits protocols. Regular audits help spot discrepancies in data and offer chances for fixes before analyzing data. Routine audits enhance reliability by 15%, which brings confidence in insights from the data.
By applying best practices for robust data collection, such as standardizing entry processes, using automation, and performing audits, organizations can improve data reliability and quality. Strong aspects allow for better integration of advanced technologies like AI to boost the data collection even more.
Integrating AI for Enhanced Data Collection
In a world driven by data, integrating artificial intelligence (AI) in collecting information reshapes how organizations gather and analyze it. Businesses gain efficiency and accuracy when they employ AI technologies for their data collection efforts. This shift leads to robust data collection strategies and better decision-making.
One major benefit gained from AI in data collection is its ability to reach hard-to-access demographics. For example, Yazi uses AI to pull insights from population segments which regular methods might miss. This is vital for gathering comprehensive and representative data, fostering informed strategies based on various perspectives.
Also, using real-time analytics in AI allows organizations to receive instant feedback on the data's relevance and accuracy. Continuously analyzing data streams helps identify discrepancies and biases as they happen, ensuring decisions rely on accurate information. This aspect greatly contributes to robust data collection by marking issues swiftly for methodological adjustments.
Some notable cases show that AI-driven collection methods have boosted user engagement. Firms that leverage AI to hone outreach strategies experience better response rates and improved interactions. This enhanced engagement results in more dependable datasets, which support robust data collection practices crucial for actionable insights.
As we look ahead, the next topic will explore diverse collection techniques and methodologies enhanced by AI's implementation. To grasp these methods is vital for ensuring that collected data meets quality and accuracy standards necessary for robust data collection.
Data Collection Techniques and Methodologies
Robust data collection is essential in business analytics. It directly affects the quality of insights for analytical purposes. Using robust data collection techniques ensures gathered information is accurate and relevant. This provides a solid base for decision-making. Here are several effective data collection methods often used:
1. Surveys and Questionnaires: Surveys are a popular way to collect data from a big audience. Theres flexibility in online or in-person formats. Yet, biases from participants and flaws in the survey design can affect effectiveness. A well-done survey can achieve a response rate of up to 70%, giving a larger dataset for analysis.
2. Interviews: One-on-one interviews can provide deep insights often missed in surveys. They let interviewers explore topics in detail. However, interviewer bias and how time-consuming this process is can be setbacks. Many leading tech firms have embraced interviews, claiming innovative insights arise from this method.
3. Focus Groups: Focus groups gather qualitative data through group discussions. This brings different views together and uncovers motivations behind consumer actions. A drawback can be dominant voices that skew the conversation. Companies depending on focus groups have launched successful products by tuning into consumer sentiments revealed in these discussions.
4. Observational Research: This method means observing subjects in their natural setting without interference. Authentic insights into customer behavior appear, especially in stores. Nonetheless, there can be limits regarding the scale and depth of data. Chains using observational methods often gain quick feedback that aids their strategies.
5. Secondary Data Analysis: Analyzing existing data allows organizations to save time and resources. But outdating can lead to complications. Making strategic decisions using secondary data can save costs and often results in better insights.
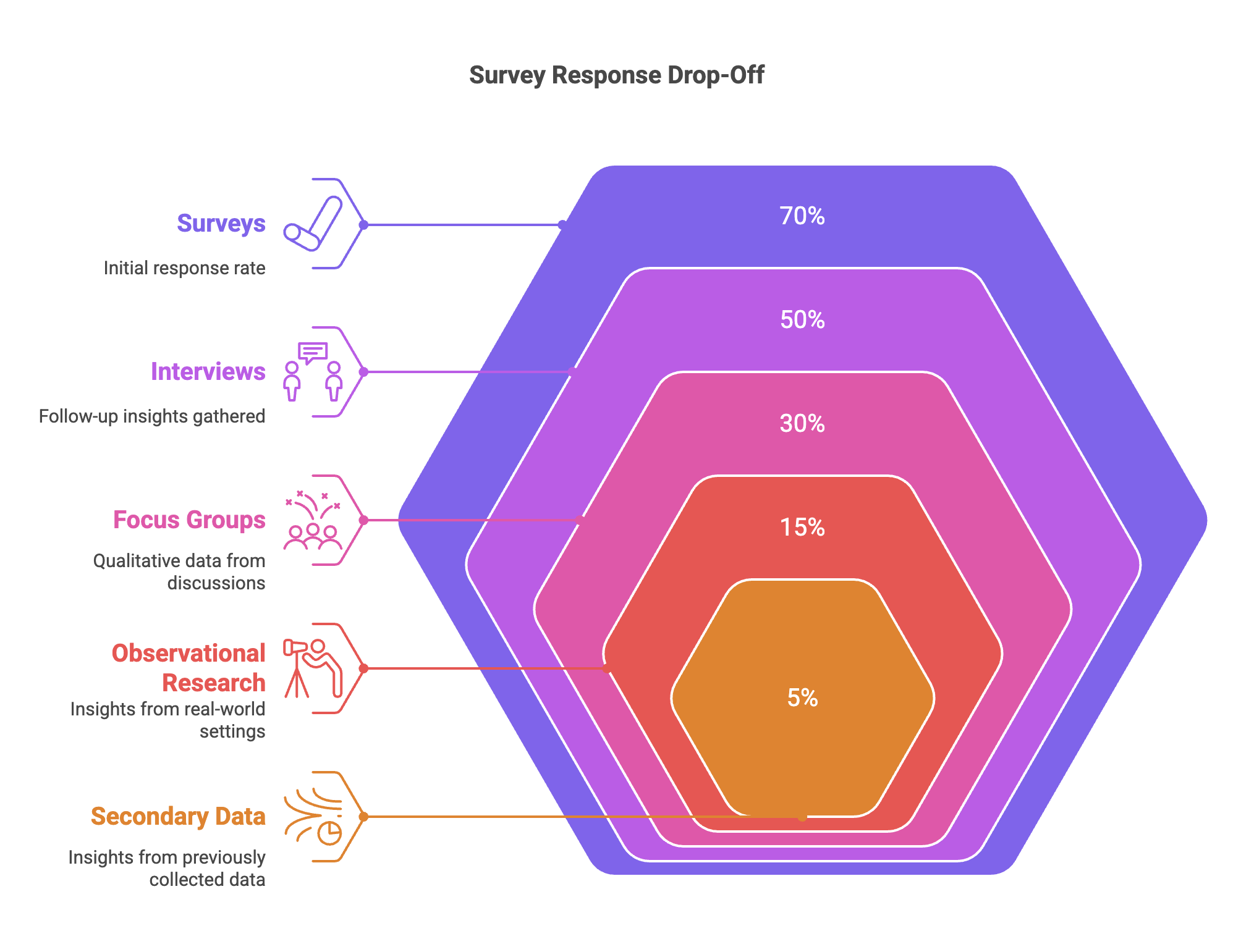
Selecting suitable methods is very important. Combining these techniques can often produce best outcomes, allowing for a triangulation of data that reduces biases from individual methods. For example, one retail company successfully combined surveys and observational research to enhance their service strategy by revealing gaps between customer expectations and reality.
In robust data collection, it’s vital for firms to use a variety of techniques for complete insights. The chosen methods should match research goals and target audience. As businesses react to market changes, a mixed approach to data collection stays essential.
After reviewing the various data collection techniques, establishing a data governance framework is important. This will boost collection methods while ensuring data quality and integrity. Decision-makers gain from accurate and actionable insights.
Data Governance Framework for Quality Control
Creating a robust data collection process is vital for organizations that seek accurate and trustworthy insights. A solid framework helps define standards, roles, and responsibilities in data management. This approach keeps data integrity intact throughout its lifecycle. It creates a path for consistent protocols that reduce risks tied to data quality problems impacting decisions.
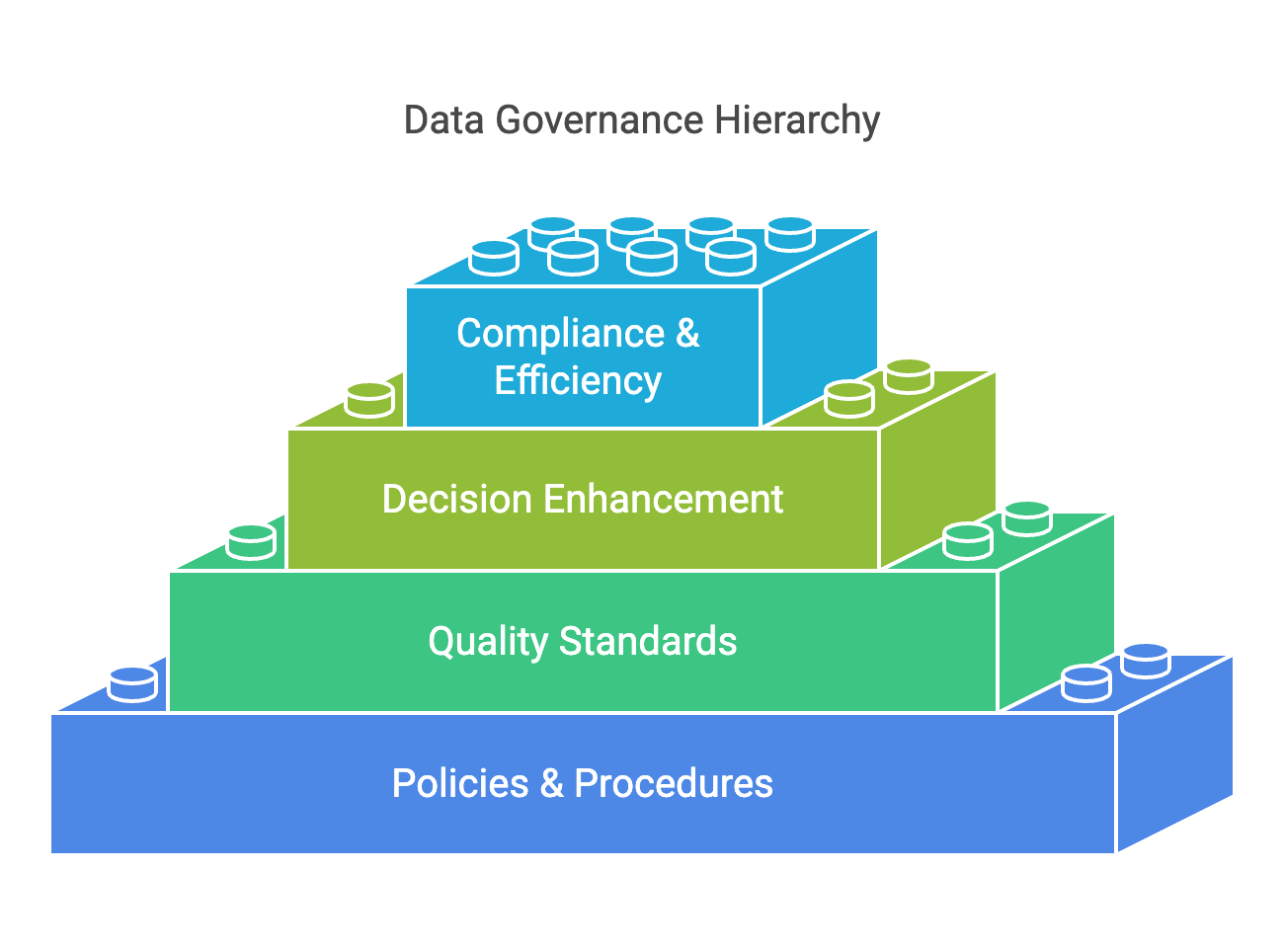
To achieve data integrity, organizations should enforce standardized handling processes. Standardization cuts down variability and makes sure all collected data follows the same quality checks. It's important to use recognized data governance practices. Documentation, audit trails, and clear entry guides are key. These approaches boost the reliability of data used in various analyses.
The importance of data stewards is crucial within the governance structure. Their job is to manage data quality and ensure adherence to governance practices. Continuous involvement helps find and address data problems early. By assigning data stewards, accountability is established. This makes it easier to identify who is responsible for any errors in data.
Many firms have benefited from solid governance frameworks. For example, those that put structured data governance in place saw gains in quality metrics of up to 80%. This number shows how effective governance can be for boosting accuracy and improving insights from data analysis.
As companies depend more on data for operations and strategies, adopting a governance framework isn't just good to do. Its a requirement. With a robust governance base, organizations can move to the next step of ensuring data quality through ongoing monitoring. This strategy doesn’t only solve present data issues but also averts possible discrepancies in the future, ensuring consistent reliability and integrity in data.
Continuous Monitoring for Data Quality Improvement
For robust data collection, a framework for continuous monitoring is key. Continuous monitoring helps to support better data quality. It allows organizations to find anomalies and issues in data quickly. This method ensures discrepancies get dealt with swiftly before they become major problems that could harm insights.
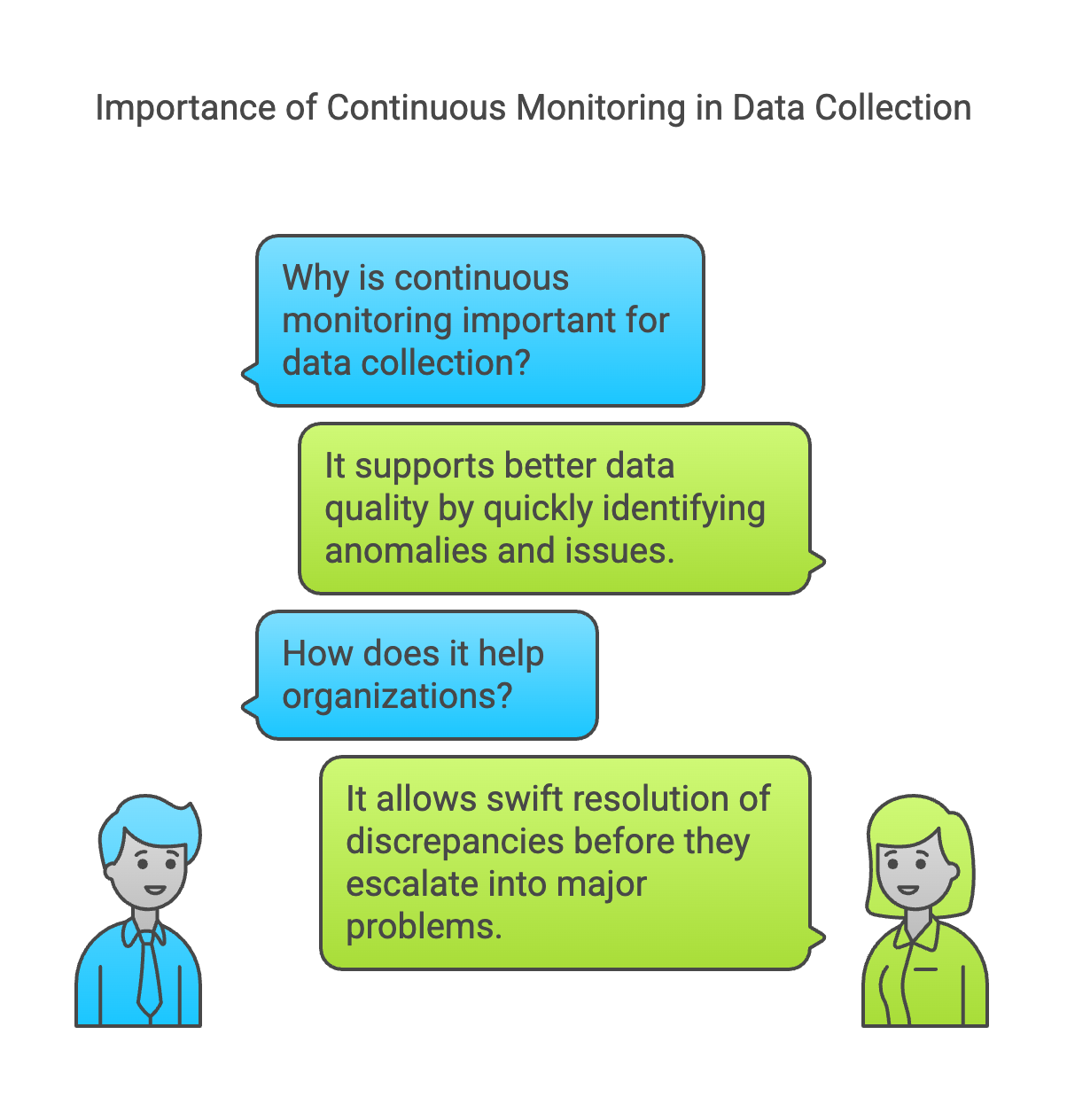
Advanced tools and tech are important for real-time data monitoring. These tools automate how data is evaluated, which help to find issues with accuracy, completeness, and consistency. When organizations use machine learning and data validation software, they can keep high data integrity in their collection process.
Creating key performance indicators for data health is an important part of constant monitoring. KPIs can measure fillers like data completeness (e.g., filled fields), consistency (e.g., formatting), and accuracy (e.g., error rates in reports). Regularly measuring these KPIs helps organizations evaluate their data health and modify their collection methods.
Benefits of proactive monitoring are huge. Quickly spotting issues allow organizations to put fixes in place faster, saving time and resources. Ongoing monitoring also builds a culture of data quality awareness in teams, making them focus on accuracy. This hard work boosts data insight reliability and improves data-driven decisions overall.
Conclusion
In the current data-driven era, ensuring robust data collection is crucial for accurate insights that inform decision-making. This article discussed practices like standard methods, AI tool integration, and a solid data governance framework. Adopting these strategies helps business quality and offers a competitive edge.
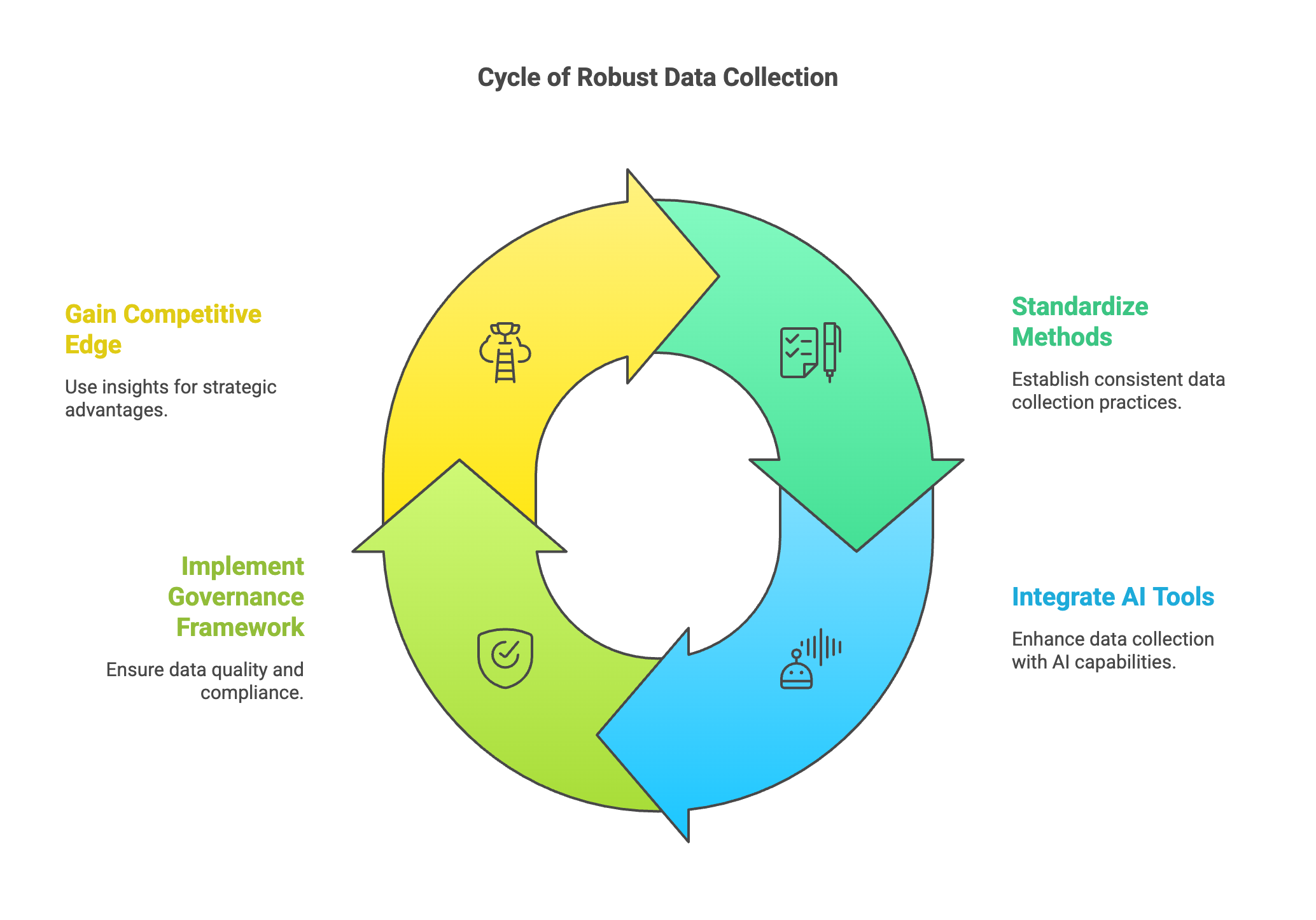
Now is the time to take steps towards enhancing your data collection processes. Use the techniques we outlined, consistently check your data integrity, and adjust approaches where necessary. Keep in mind that the quality of insights ties directly to the strength of your data collection efforts. The effect of properly collected data is significant for business outcomes.
By focusing on robust data collection, you empower your organization to make sound decisions and achieve real results. Implement these insights today and and see your data capabilities improve fast!
About Yazi
Yazi offers an innovative market research platform that leverages WhatsApp to facilitate high-response surveys, diary studies, and in-depth interviews, providing businesses with rich qualitative and quantitative insights.
This matters because Yazi's unique approach enables researchers to connect with hard-to-reach demographics through familiar digital channels, ultimately enhancing research efficiency and engagement.
Discover how Yazi can transform your market research today!