Introduction
Unraveling the treasure of insights that open-ended questions hold can feel like a daunting task. But fear not, because we've got a systematic and comprehensive 5-step guide to help you tackle this with ease. From data importing to refining your analysis, our approach is designed to make the process of analyzing open-ended questions both efficient and insightful. Dive in, and uncover the gems of wisdom that your WhatsApp research data holds!
Step 1: Import Data Into our Template
(Click here to get access to our template)
1) Export any data and import it to the template. These going to be the answers that would have collected from your WhatsApp research.

2) Paste your Open Text Responses into the "Text to Analyse" Box
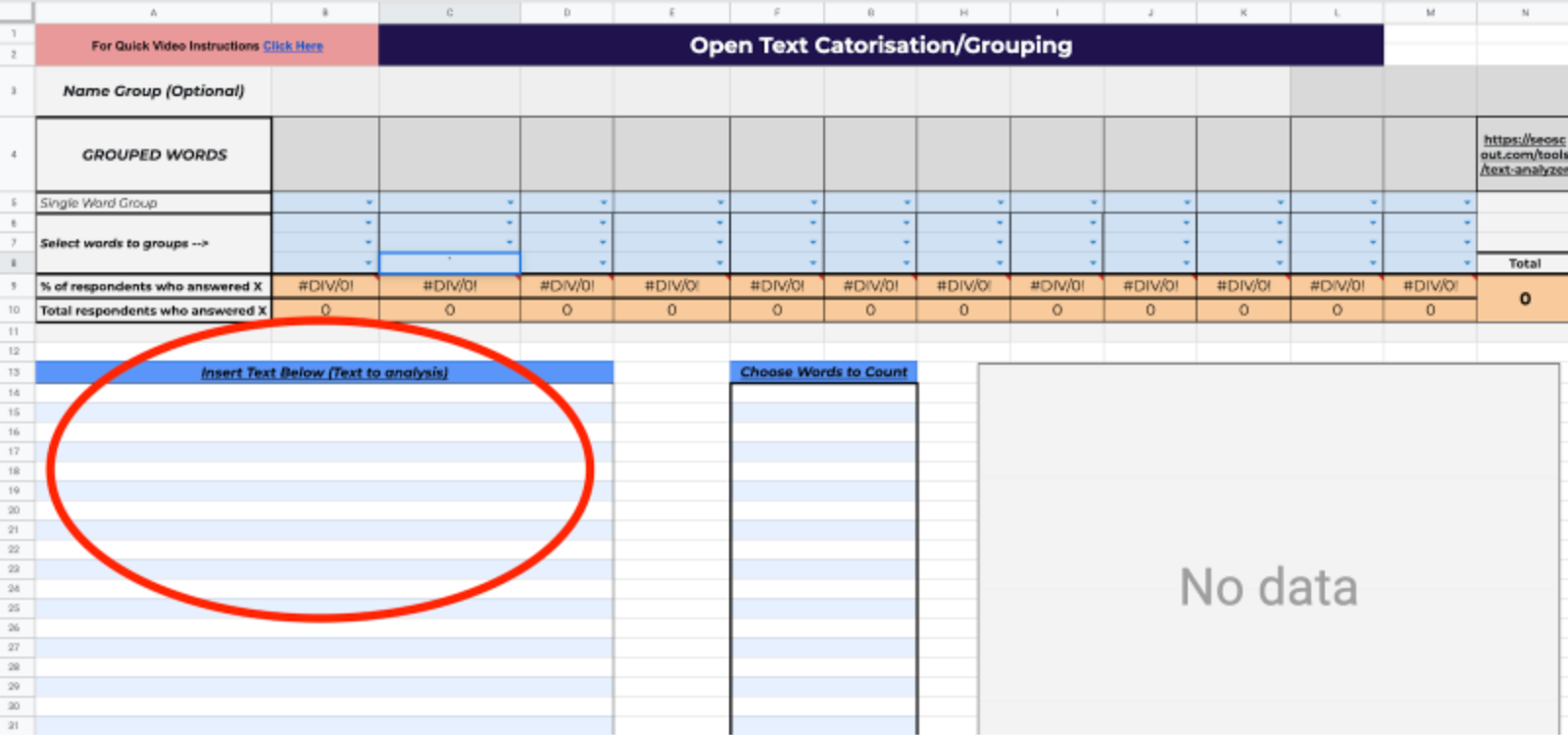
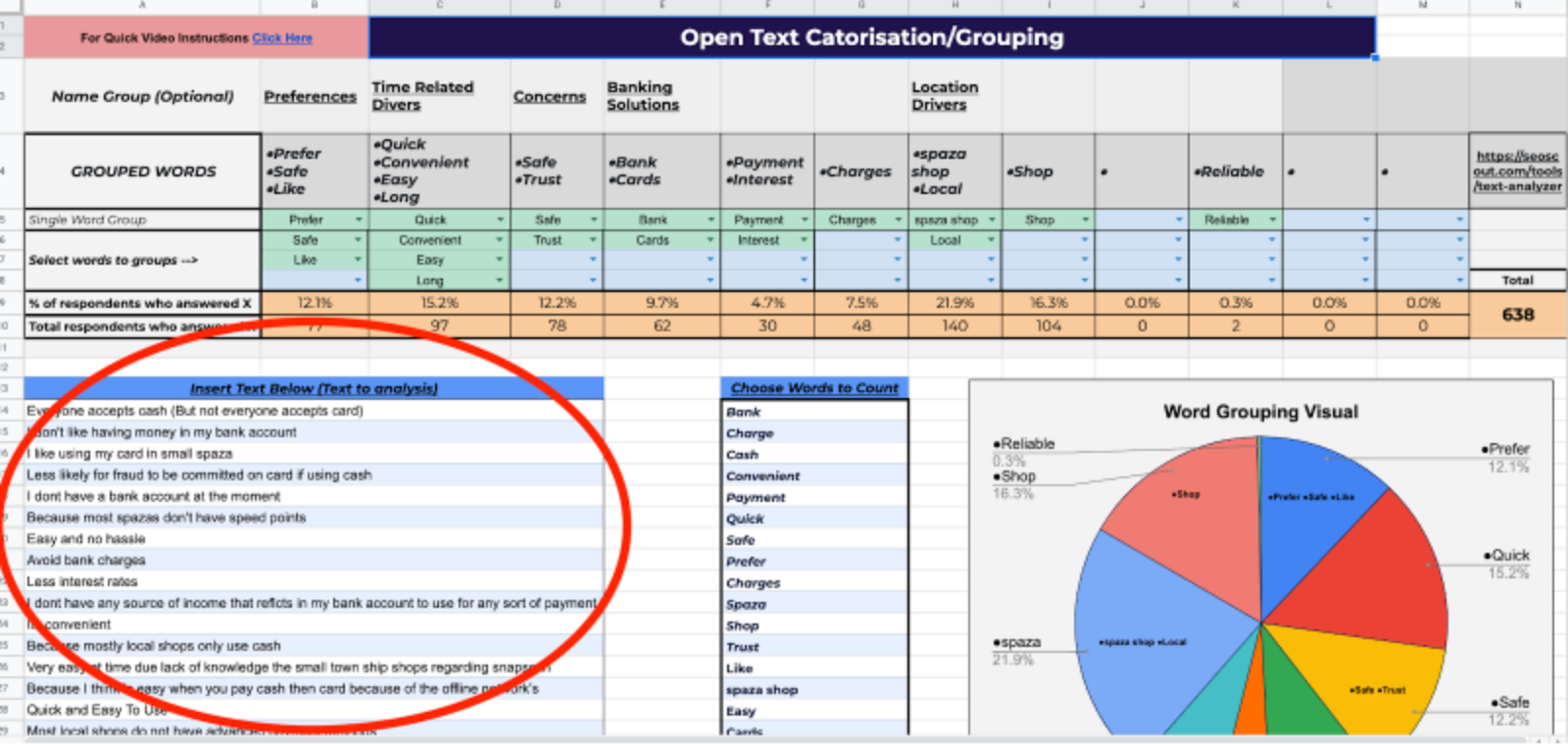
3) Choose the Words you would like to Group/count. Type them below "Chose Words to count"
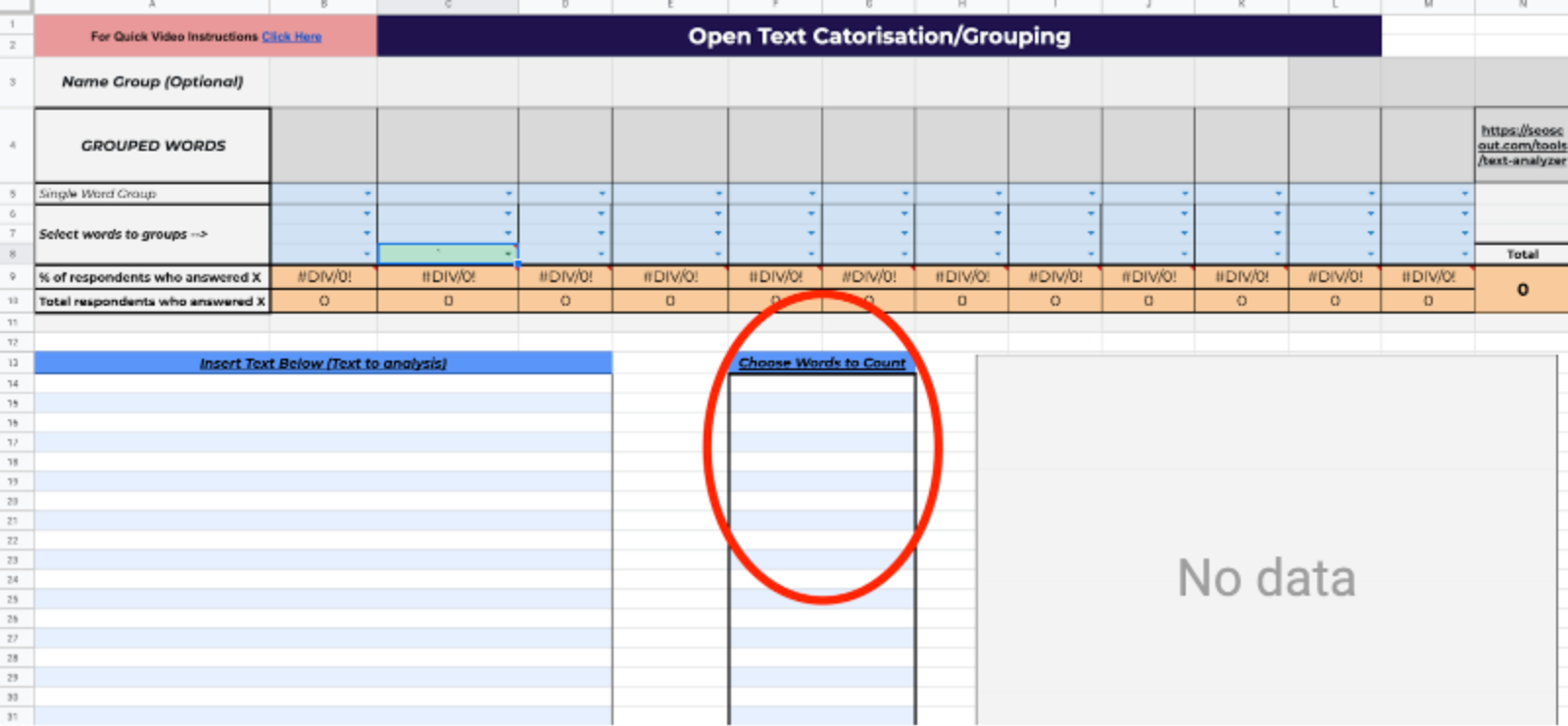
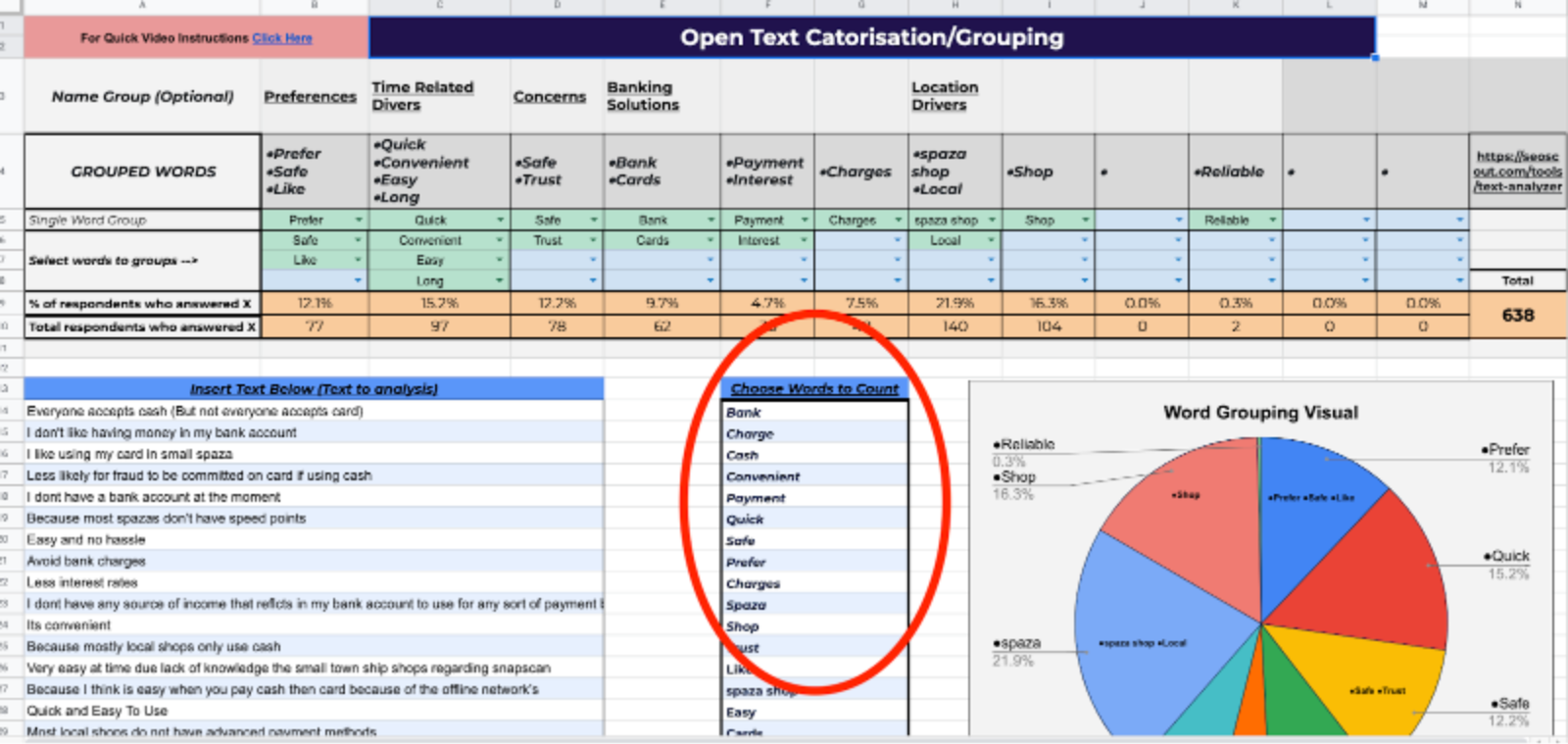
Step 2: Identify Response Categories
A response category is a set of answers given by respondents that are around the same topic. For example in the sample data, we have example categories like "Bank", "Cash" etc
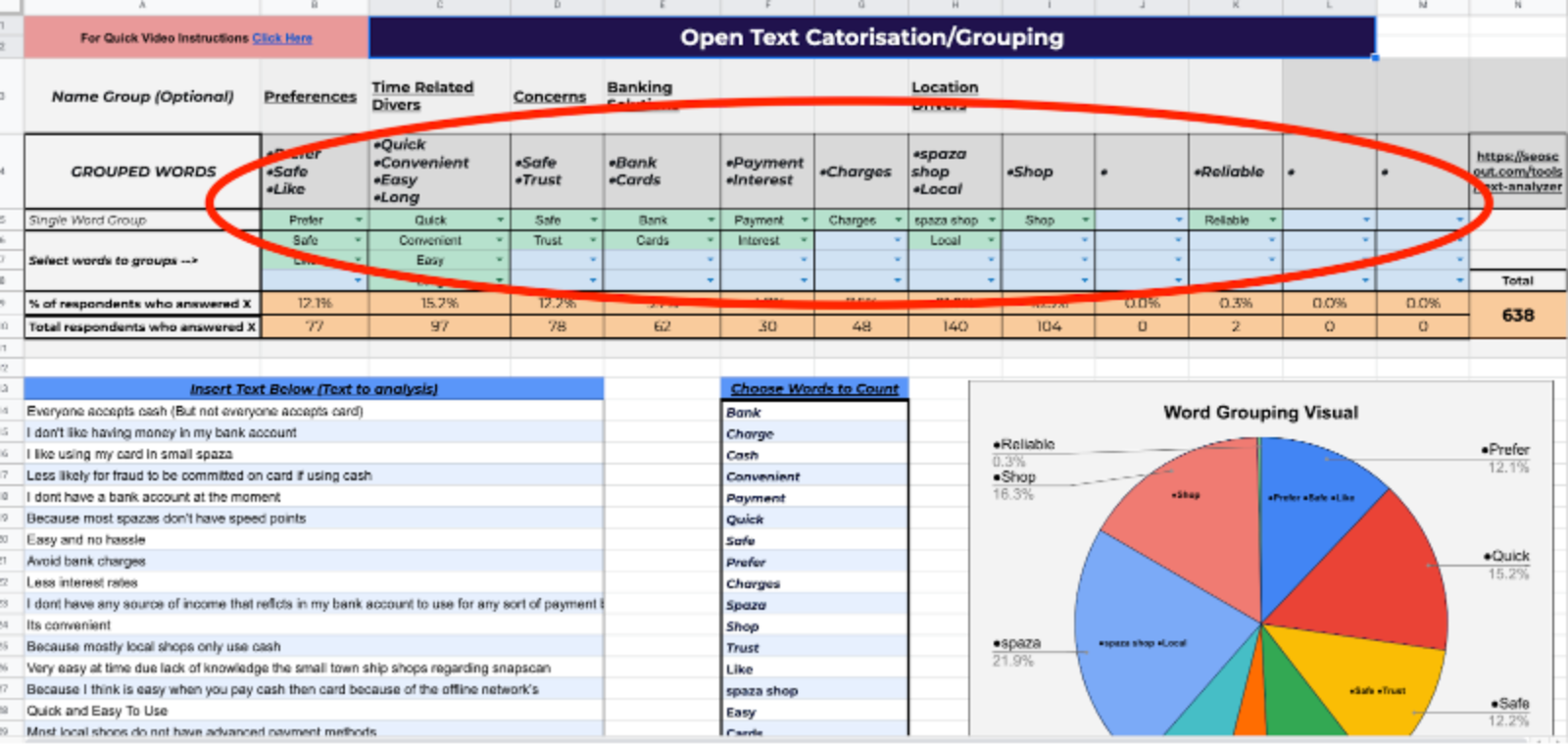
In theory, you could go through every answer to identify your response categories one-by-one, but that wouldn’t be very efficient. Instead, we’re going to use a series of techniques that help you identify the broad categories.
A) Use a Text Analyser: text analysers take your data and analyse it for the most commonly used words in your text, which helps you identify broad categories of responses.
Here's a tool that can help you with that!
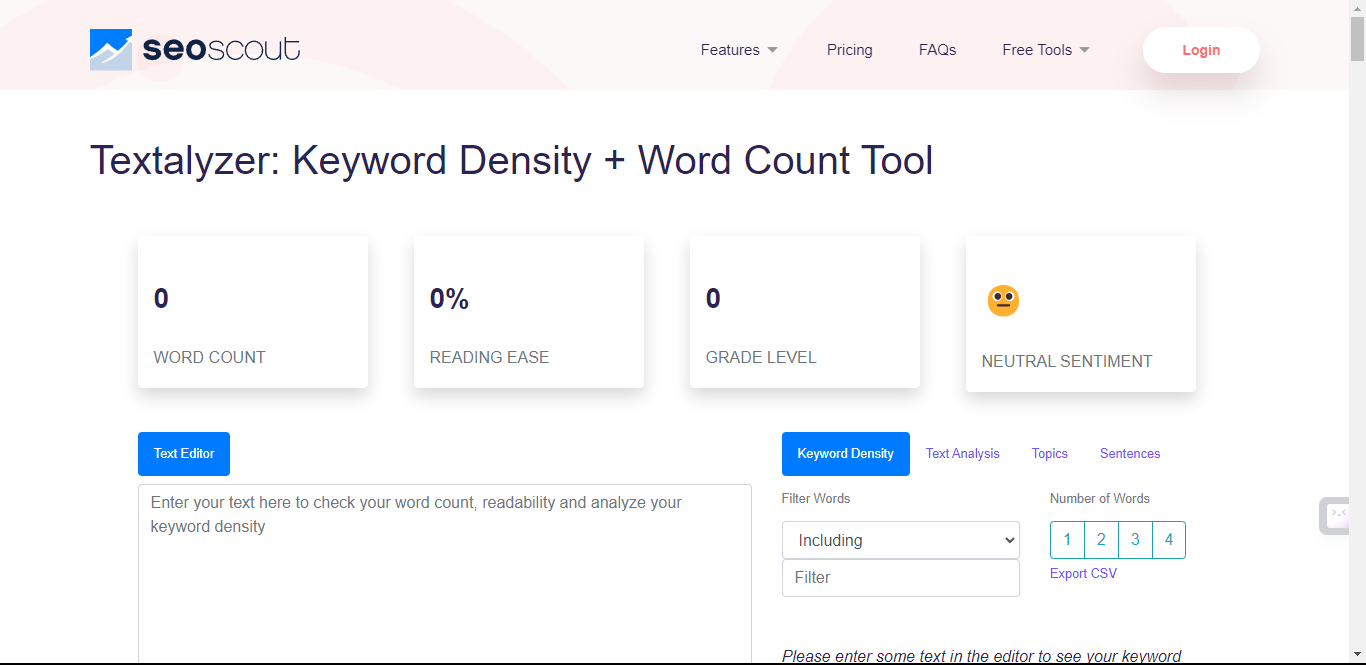
All you have to do is paste the open text answers into the text editor box and you'll be able to filter through the frequency of any specific word in your open text answers.
As such, they represent some of the most popular replies to the question we asked. They don’t represent all the answers, of course, but they’re a good place to start when building the list of response categories.
Add each category to the top of separate a separate column like in the example above:
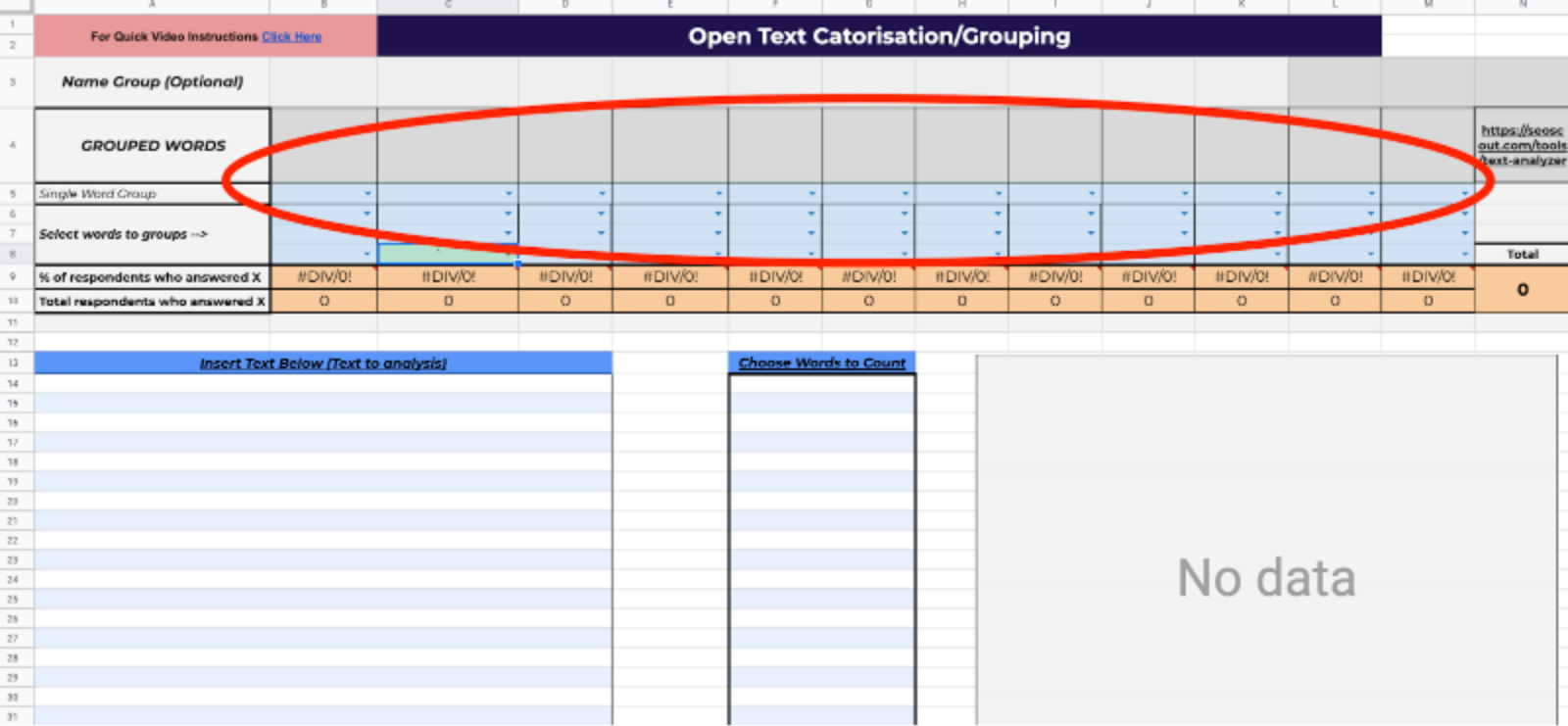
How To Create Response Categories using ChatGpt:
To begin the analysis, we can manually read through the survey responses and identify common themes. This step helps us create a set of categories that encompass the different factors mentioned. In our example, we have identified the following categories: corruption, equipment failure, maintenance issues, capacity limitations, non-payment impact, fuel shortage, and political interference, among others.
Using GPT Prompts for Categorisation: Now comes the exciting part – leveraging the power of GPT prompts to automate the categorisation process. We can input each survey response into the GPT model and use a prompt that asks it to categorise the response into the relevant topic.
For this example we are going to be using some of the data from our load-shedding report :
Prompt: Please categorise these responses into one or more topics:
"The government owes other countries."
"Bad management and corruption"
"Generators keeps breaking down"
It's important to note that the quality of the categorisation depends on the model's training data and its understanding of the prompt.
Response
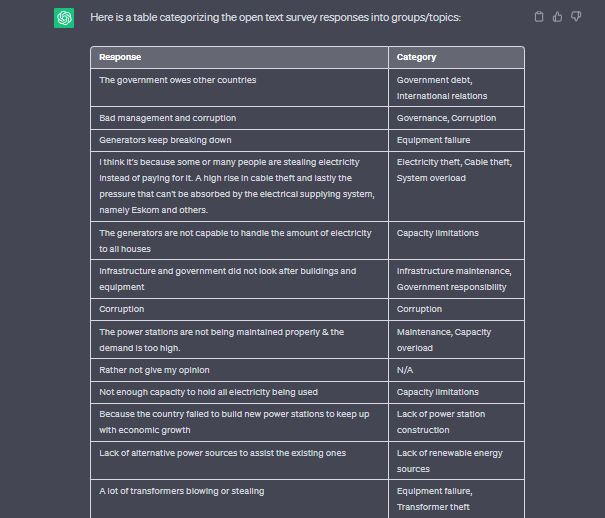
Tabulating the Results
After categorising all the responses using GPT prompts, we can create a table that displays the frequency and percentage of each topic mentioned. This table provides a clear overview of the major themes identified in the survey responses. By focusing on the topics with higher counts and percentages, we can prioritise addressing those concerns.
Prompt: Create a table of how many times each topic was mentioned. Column A is the topic, Column B is the count of the times the topic was mentioned, Column C is the percentage of the total survey responses

This is how it will look
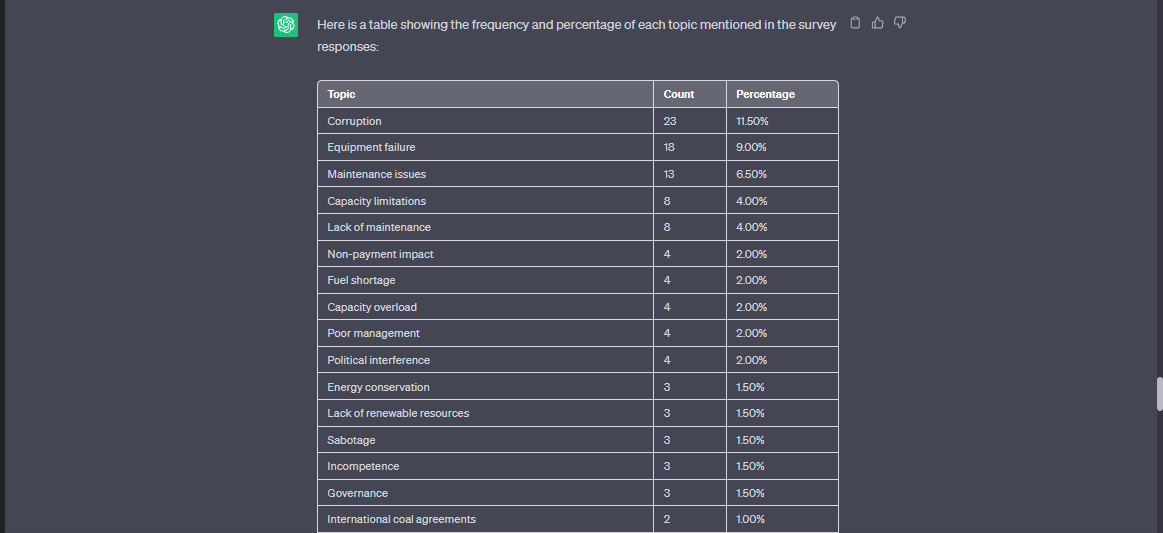
Step 3: Record the Responses for each category and
1) Place a '1' in each cell where a response (the row) matches a category (the column) to identify a positive response in each category. Add categories as you go.
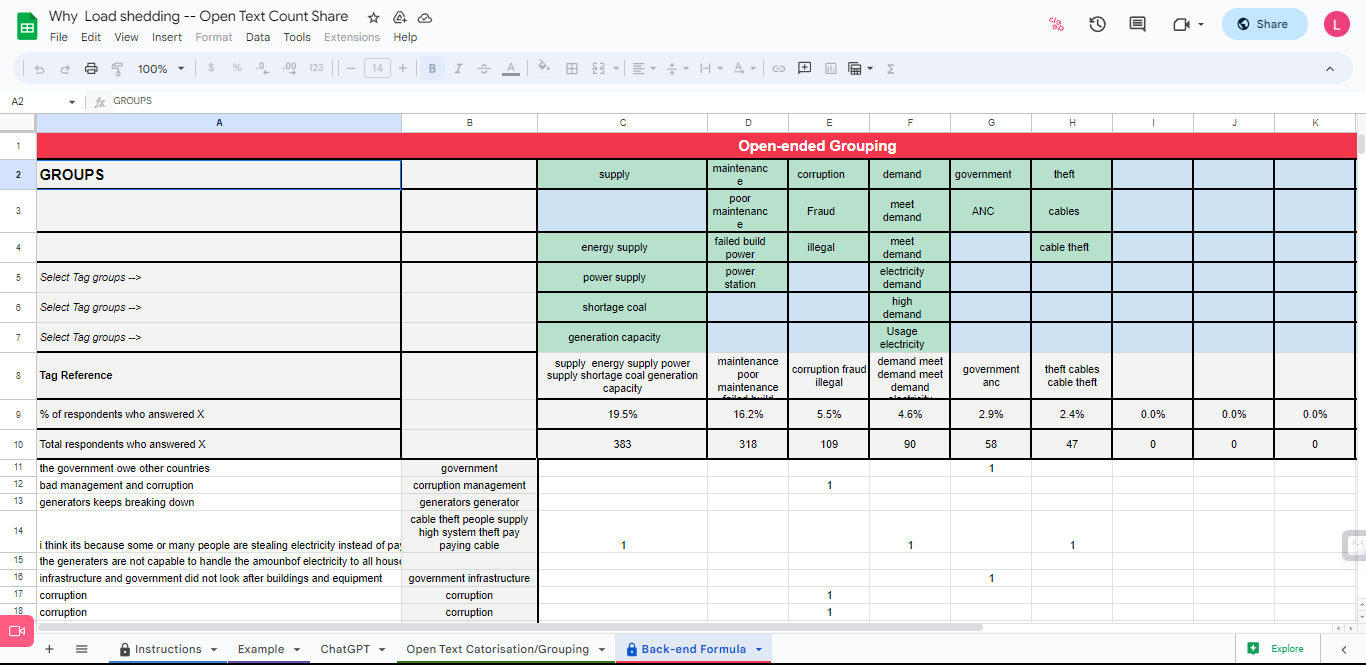
2) Robust Word Grouping Visual : After you have filled in all of your data, you will be given a visual that depicts each of the groups you have made in a pie chart.
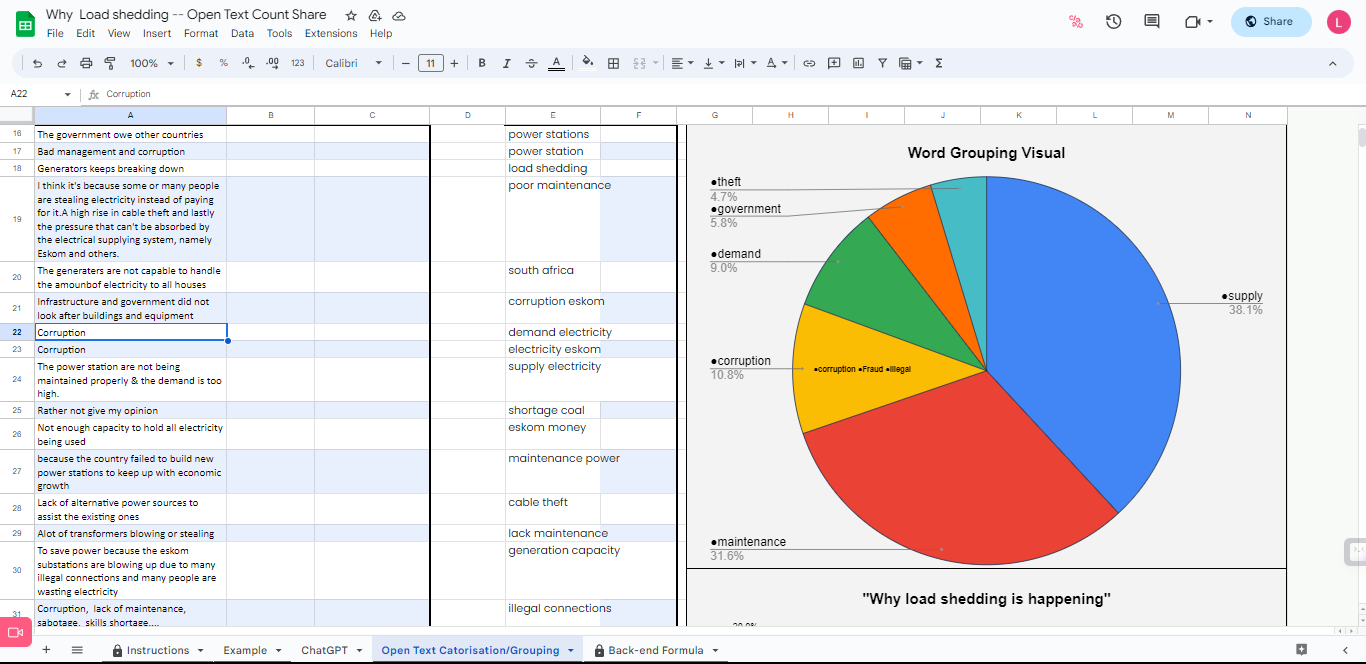
Best Practices
Efficient Data Importing:
- Use the provided template for an organized approach.
- Export and import your WhatsApp research data into the template for ease of analysis.
Categorizing Responses:
- Identify response categories by examining common themes in the answers.
- Utilize tools like text analyzers (e.g., seoscout.com/tools/text-analyzer) to pinpoint frequently used words, aiding in categorization.
- Add categories to your analysis template to maintain organization.
Creating Response Categories:
- Manually read through responses to identify common themes.
- Use these themes to create a comprehensive set of categories.
- Consider categories like "corruption," "equipment failure," or "political interference" depending on your survey topic.
Utilizing GPT Prompts for Automated Categorization:
- Leverage GPT prompts to categorize each response into relevant topics efficiently.
- Ensure the prompts are clear and specific to improve categorization accuracy.
Tabulating Results for Clarity:
- After using GPT for categorization, create a table displaying the frequency and percentage of each topic mentioned.
- Focus on topics with higher counts for a better understanding of prevalent issues or opinions.
Recording Responses in Each Category:
- Mark '1' in cells where a response matches a category in your template.
- This systematic approach helps in identifying which responses fall under which category.
Visual Representation of Data:
- Utilize the template’s feature to generate a visual representation, like a pie chart, showing the distribution of grouped words.
- Visuals aid in better understanding and presenting your analysis.
Combining Manual and Automated Methods:
- Blend manual categorization with GPT prompts for a balanced approach.
- This combination enhances both the depth and efficiency of your analysis.
Accuracy and Reliability:
- Remember that the quality of categorization depends on the model's training and the precision of your prompts.
- Regularly review and adjust your approach for accuracy.
Conclusion
By combining manual categorisation with the power of language models like GPT, we can save time and resources while obtaining valuable information. However, it's important to remember that the accuracy of the categorisation relies on the quality of the prompt and the model's training data. With this newfound knowledge, you can confidently approach open-ended question analysis using GPT prompts and unlock valuable insights from your survey data.
Remember, practice makes perfect, so don't hesitate to experiment with different prompts and refine your approach to suit your specific dataset and research objectives. Happy analysing!
FAQ
How can researchers ensure the accuracy of ChatGPT's categorization of open-ended responses?
Ensuring accuracy involves validating ChatGPT's output through random sampling and manual checks, adjusting categorization criteria based on expert review, and continuously refining the model with feedback.
What are the limitations of using ChatGPT for analyzing open-ended survey responses?
Limitations include potential bias in AI training data, difficulty in understanding context or nuance, and the need for manual oversight to ensure relevance and accuracy.
How can the analysis of open-ended questions with ChatGPT be scaled for larger datasets?
Scaling the analysis for larger datasets can be achieved by automating data preprocessing, using batch processing for analysis, and iteratively refining the categorization template based on initial outcomes